Multimodal Agent Orchestration: Integrating Vision, Audio, and Text Processing
Multimodal agent orchestration has emerged as a critical framework for creating AI systems that can perceive, understand, and interact with the world in ways that more closely mirror human cognition
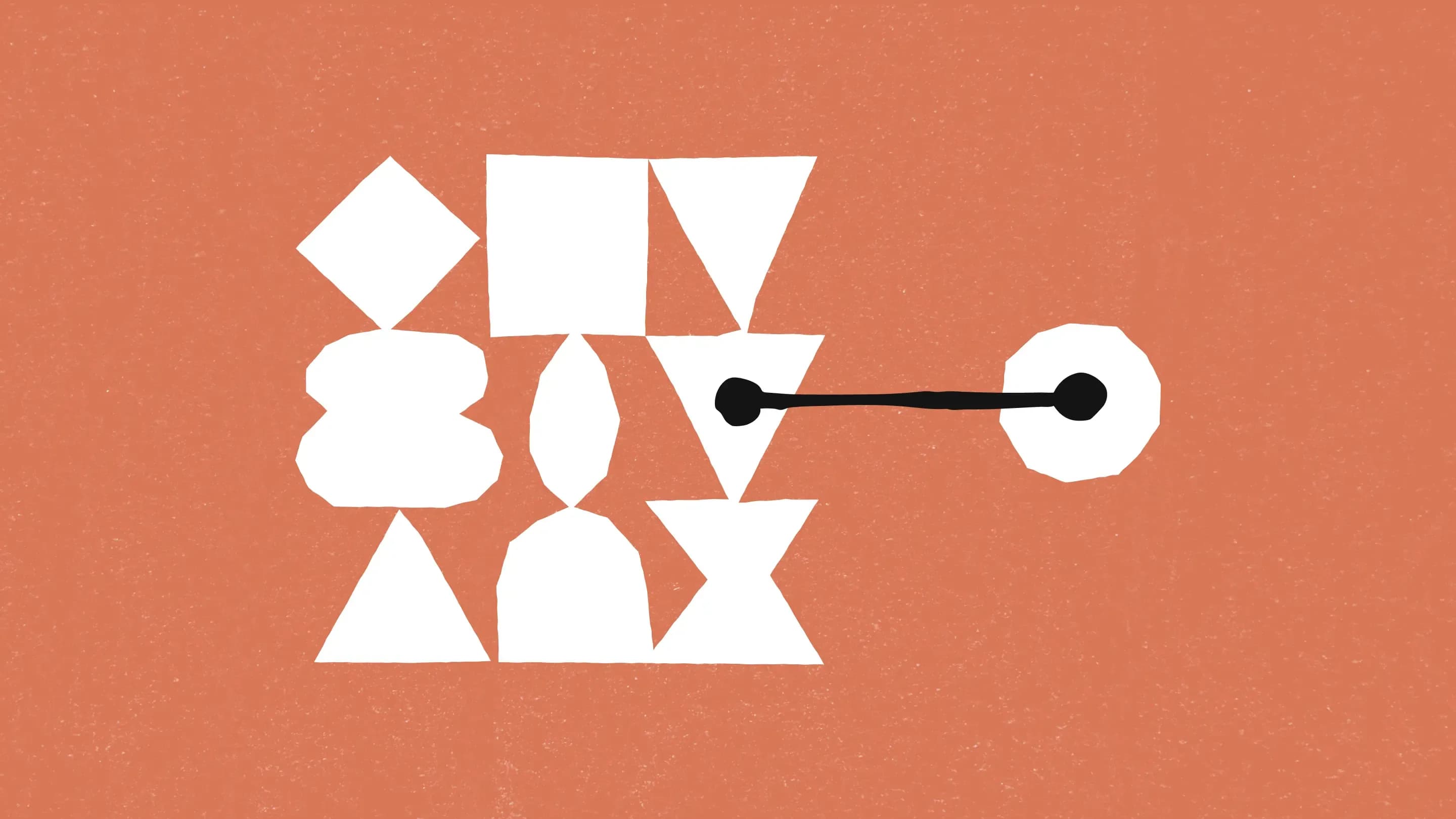
This approach transcends the limitations of single-modality AI by enabling systems to process and synthesize information across different sensory channels, creating more robust, versatile, and intuitive AI experiences.
What Is Multimodal Agent Orchestration?
Multimodal agent orchestration refers to the systematic coordination of AI agents that process different data types (text, images, audio) to perform complex tasks requiring cross-modal understanding. Unlike traditional unimodal systems that operate in isolation, multimodal orchestration creates a harmonized framework where specialized agents collaborate, share insights, and produce integrated responses.
Key Components:
- Specialized Agents: Dedicated models optimized for specific modalities (vision models, speech recognition systems, NLP engines)
- Coordination Layer: Middleware that manages information flow between agents
- Cross-Modal Reasoning Engine: Systems that integrate insights from different modalities
- Unified Response Generation: Mechanisms that produce coherent outputs drawing from multiple agents
The Business Value of Multimodal Integration
Organizations implementing multimodal agent systems can achieve significant competitive advantages:
- Enhanced Customer Experience: More natural and intuitive human-computer interactions
- Improved Decision Making: Access to richer, more comprehensive data analysis
- Operational Efficiency: Automation of complex tasks requiring multiple sensory inputs
- New Product Opportunities: Creation of innovative applications impossible with single-modality AI
According to recent market analysis, businesses deploying multimodal AI solutions report a 37% increase in customer satisfaction and a 28% improvement in operational efficiency compared to traditional AI implementations.
Technical Architecture for Multimodal Systems
Foundation Models and Specialization
Modern multimodal systems typically build upon foundation models that have been pre-trained on vast datasets across modalities. These models often employ:
- Joint Embedding Spaces: Unified vector representations that align concepts across modalities
- Cross-Attention Mechanisms: Allowing one modality to query information from another
- Modal-Specific Encoders: Specialized processing pathways for each input type
- Shared Reasoning Layers: Common processing systems that operate on unified representations
Orchestration Frameworks
The orchestration layer serves as the conductor of this multimodal symphony, managing:
- Input Routing: Directing data to appropriate specialized agents
- Information Exchange: Facilitating communication between agents
- Execution Sequencing: Determining the order of operations
- Conflict Resolution: Reconciling contradictory outputs from different agents
- Response Synthesis: Integrating insights to produce cohesive outputs
Real-World Applications
Healthcare Diagnostics
Multimodal systems in healthcare combine:
- Visual analysis of medical imaging (X-rays, MRIs)
- Processing of patient verbal descriptions
- Integration of text-based medical records
- Analysis of audio biomarkers (breathing patterns, voice characteristics)
These integrated systems achieve diagnostic accuracy rates up to 18% higher than single-modality approaches.
Advanced Customer Service
Modern customer service platforms leverage multimodal orchestration to:
- Process customer images of products
- Understand verbal descriptions of issues
- Analyze text-based chat histories
- Recognize emotional cues from voice
- Generate appropriate responses across channels
Content Understanding and Creation
Media companies employ multimodal systems to:
- Analyze visual elements in videos
- Transcribe and understand spoken content
- Process textual metadata
- Generate comprehensive content summaries
- Create multimodal content recommendations
Implementation Strategies
Phased Approach to Multimodal Integration
Organizations can adopt a structured implementation pathway:
- Assessment: Identify high-value use cases for multimodal integration
- Agent Selection: Choose or develop specialized agents for each modality
- Orchestration Design: Create the coordination framework
- Pilot Implementation: Deploy in controlled environments
- Iterative Refinement: Continuously improve based on performance data
Technical Considerations
When implementing multimodal systems, organizations should consider:
- Computational Requirements: Multimodal systems often demand significant processing power
- Latency Management: Orchestration must minimize delays between agent interactions
- Scaling Considerations: Architecture should support growth in both data volume and usage
- Fallback Mechanisms: Systems need graceful degradation when modalities are unavailable
Challenges and Solutions
Integration Complexity
Challenge: Aligning representations across fundamentally different data types.
Solution: Developing robust joint embedding spaces and implementing cross-modal attention mechanisms that allow representations to inform each other.
Computational Efficiency
Challenge: Running multiple specialized models simultaneously can be resource-intensive.
Solution: Implementing model distillation, quantization, and on-demand agent activation to optimize resource usage.
Data Requirements
Challenge: Obtaining aligned multimodal training data is difficult and expensive.
Solution: Leveraging self-supervised learning techniques and synthetic data generation to expand training datasets.
The Future of Multimodal Agent Orchestration
As we look toward the future, several trends will shape the evolution of multimodal systems:
Emergent Intelligence
Future multimodal systems will likely demonstrate greater emergent capabilities—abilities that aren't present in any individual component but arise from their interaction. This suggests possibilities for more creative problem-solving and adaptive learning.
Seamless Multimodal Interaction
The distinction between modalities will blur from the user perspective, creating truly natural interfaces that select the appropriate modality based on context and user needs.
Personalized Agent Ecosystems
Organizations will develop customized agent ecosystems tailored to specific domains, with specialized agents that reflect unique business knowledge and processes.
Conclusion
Multimodal agent orchestration represents a fundamental shift in AI system architecture, moving from isolated, specialized models to integrated ecosystems of collaborating agents. For businesses seeking to leverage the full potential of artificial intelligence, multimodal integration offers a path to more powerful, flexible, and human-like AI capabilities.
By thoughtfully implementing orchestration frameworks that coordinate vision, audio, and text processing agents, organizations can unlock new levels of AI performance and create experiences that more naturally bridge the gap between human and machine intelligence.
Get Started with Multimodal AI
Ready to explore how multimodal agent orchestration can transform your business? Contact our team of AI integration specialists to discuss your unique use case and discover the potential of unified vision, audio, and text processing for your organization.
Start Building AI Workflows Today
Launch for free, collaborate with your team, and scale confidently with enterprise-grade tools.